This talk presents advances towards the development of effective projection-based reduced order models (ROMs) for complex multi-scale multi-physics problems. As a representative application, we consider combustion dynamics in a rocket engine, which is characterized by the coupling between heat release, hydrodynamics and acoustics.
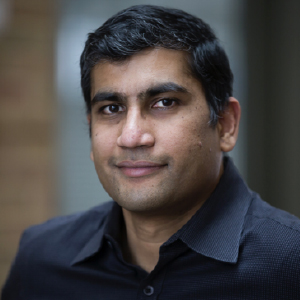
The first part of the talk is focused on improving robustness and consistency: A structure-preserving transformation of the state variables is used along with a discretely consistent least squares formulation to yield symmetrized model operators in both explicit and implicit time integration settings. The resulting reduced order model is well-conditioned and globally stable. Local stability is promoted via limiters that enforce physical realizability.
The second part of the talk is focused on improving accuracy: Dimension reduction approaches based on static linear manifolds are not effective in addressing multi-scale problems with significant convection. To help mitigate this issue, we present formulations to adaptively choose the projection basis and sampling points. The last part of the talk discusses tractability : ROMs are used to enable computations of problems for which full order models are not affordable. In particular, we develop a multi-fidelity framework in which component-level ROMs are trained on small domains, and integrated to enable full-system predictions in an affordable manner. In essence, geometry-specific training is replaced by the response generated by perturbing the characteristics at the boundary of the truncated domain. This training method is shown to enhance predictive capabilities and robustness of the resulting ROMs, including conditions outside the training range.
Karthik Duraisamy is an Professor of Aerospace Engineering and the director of the center for data-driven computational physics at the University of Michigan, Ann Arbor.
He holds a Ph.D. in Aerospace Engineering and a Master’s in Applied Mathematics from the University of Maryland. His research interests are in various aspects of computational modeling including data-driven and reduced order modeling, statistical inference, numerical methods and physics-based modeling. He is also a founder and chief scientist of the silicon-valley-based startup Geminus.AI, which is focused on developing digital twins for industrial processes.