The motor-driven intracellular transport plays a crucial role in supporting a neuron cell’s survival and function, with motor proteins and microtubule (MT) structures collaborating to promptly deliver the essential materials to the right location in neuron. The disruption of transport may lead to the onset of various neurodegenerative diseases. Therefore, it is essential to study how neurons regulate the material transport process and have a better understanding of the traffic jam formation.
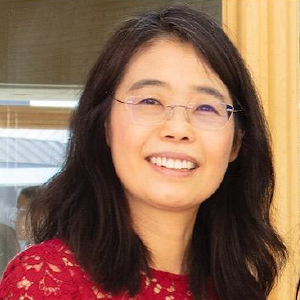
In our earlier work, we developed a PDE-constrained optimization model and an isogeometric analysis (IGA) solver to simulate traffic jams induced by MT reduction and MT swirl. Here, we develop a novel IGA-based physics-informed graph neural network (PGNN) to quickly predict normal and abnormal transport phenomena such as traffic jam in different neuron geometries. In particular, the proposed method learns from the IGA simulation of the intracellular transport process and provides accurate material concentration prediction of normal transport and MT-induced traffic jam.
The IGA-based PGNN model contains simulators to handle local prediction of both normal and two MT-induced traffic jams in pipes, as well as another simulator to predict normal transport in bifurcations. Bézier extraction is adopted to incorporate the geometry information into the simulators to accurately compute the physics informed loss function with PDE residuals. Moreover, a GNN assembly model is adopted to tackle different neuron morphologies by assembling local prediction into the entire geometry.
In summary, the well-trained model effectively predicts the distribution of transport velocity and material concentration during traffic jam and normal transport with an average error less than 10% compared to IGA simulations. The effectiveness of the proposed model is demonstrated within several complex neuron geometries.
Jessica Zhang is the George Tallman Ladd and Florence Barrett Ladd Professor of Mechanical Engineering at Carnegie Mellon University with a courtesy appointment in Biomedical Engineering.
She received her B.Eng. in Automotive Engineering, and M.Eng. in Engineering Mechanics from Tsinghua University, China; an M.Eng. in Aerospace Engineering and Engineering Mechanics, and a Ph.D. in Computational Engineering and Sciences from Institute for Computational Engineering and Sciences (now Oden Institute), The University of Texas at Austin.
Her research interests include computational geometry, isogeometric analysis, finite element method, data-driven simulation, image processing, and their applications in computational biomedicine, materials science and engineering.
Zhang has co-authored over 200 publications in peer-reviewed journals and conference proceedings and received several Best Paper Awards. She published a book entitled “Geometric Modeling and Mesh Generation from Scanned Images” with CRC Press, Taylor & Francis Group.
Zhang is the recipient of a Simons Visiting Professorship from Mathematisches Forschungsinstitut Oberwolfach of Germany, US Presidential Early Career Award for Scientists and Engineers, NSF CAREER Award, Office of Naval Research Young Investigator Award, and USACM Gallagher Young Investigator Award.
At CMU, she received the David P. Casasent Outstanding Research Award, George Tallman Ladd and Florence Barrett Ladd Professorship, Clarence H. Adamson Career Faculty Fellow in Mechanical Engineering, Donald L. & Rhonda Struminger Faculty Fellow, and George Tallman Ladd Research Award. She is a Fellow of AIMBE, ASME, SMA, USACM and ELATES at Drexel. She is the Editor-in-Chief of Engineering with Computers.